Simulation-Based Credit Analytics: Managing Tail Risk and Uncertainty
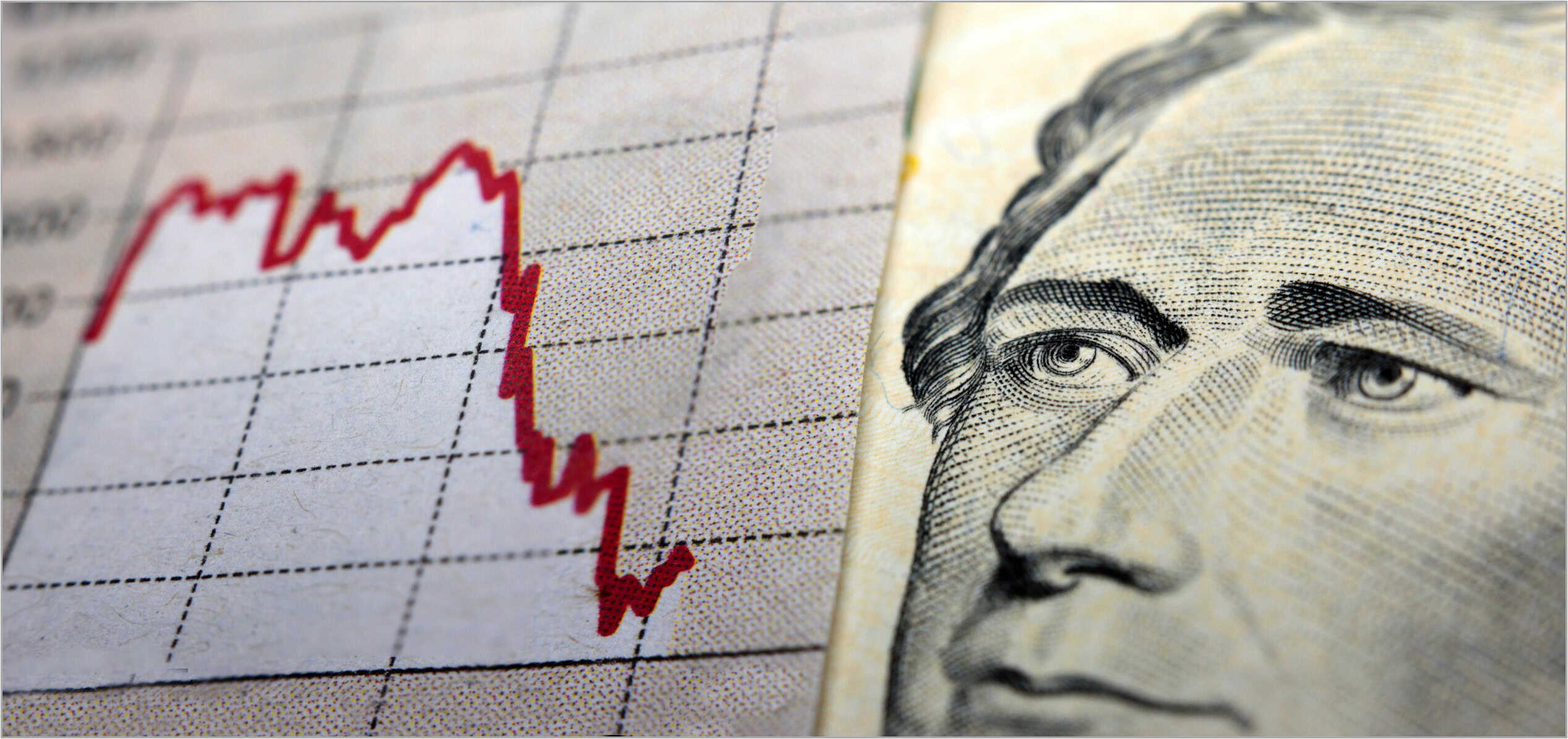
A Better Approach to Financial Services Credit Loss and Performance Forecasting
There are times when historical data is not sufficiently predictive. Certainly, the pandemic is an example of a sudden change causing historical credit data to lack predictability. Russia’s recent Ukraine attack is another. Also, future trends like Environmental, Social, and Governance (“ESG”) are expected to have a significant impact on lending volumes, credit risk, and profitability. Yet no one knows exactly how and when ESG will impact Financial Services businesses. Changes like these are reverberating through our economy with many unknown effects. Change is only accelerating. This uncertainty tests those responsible for forecasting the future. The uncertain environment challenges those responsible for credit loss estimation and providing accurate inputs to the Allowance for Credit Loss (ACL), loan pricing, loan decisions, and a wide variety of financial services analytical needs.
This blog is organized as follows:
- Simulation-based credit analytics: Background and the impact of uncertainty on credit data
- Simulation-based credit analytics: The credit forecasting under uncertainty approach
- Simulation-based credit analytics: The Sim Solution
- Conclusion
Note: In remarks presented in 2022, OCC Acting Comptroller Michael Hsu commented on increasing tail risks.
“Russia’s invasion of Ukraine is changing the landscape for tail risks; so is the rise of crypto trading. Strong, independent risk management can help the banking system successfully navigate these risks and avoid surprises.”
Tail risks are low probability and high impact risk events. In this article, we distinguish between risk and uncertainty. We consider risk to be the “known unknown” and uncertainty to be the “unknown unknown.” Risk is significantly informed by data; uncertainty is not yet significantly informed by data. We consider tail risk as functionally equivalent to uncertainty. As such, to clearly differentiate between these very different risk gradients, we use the word “uncertainty” in much the same way “tail risk” is sometimes mentioned.
I) Simulation-Based Credit Analytics: Background and the Impact of Uncertainty on Credit Data
The uncertainty related to sudden and unexpected events has been shown, in part, to be the result of a failure of imagination.
Pearl Harbor (1941)
“We were so busy thinking through some ‘obvious’ Japanese moves that we neglected to hedge against the choice that they actually made. There is a tendency in our planning to confuse the unfamiliar with the improbable.”
– Thomas Schelling forward on a book about the Pearl Harbor attack. [1]
9/11 (2001)
“….the most important failure [concerning the 9/11 attacks] was one of imagination.”
– 9/11 Commission [2]
Financial Crisis (2007)
The 2008 Financial Crisis was caused by “high risk, complex financial products; undisclosed conflicts of interest; the failure of regulators, the credit rating agencies, and the market itself to rein in the excesses of Wall Street.”
– U.S. Senate [3]
Pandemic (2020)
“The coronavirus pandemic is a failure of imagination…It’s not just policy failures that got us to this point. It’s an inability to conceive of planetary threats.”
– Stephen Kinzer is a senior fellow at the Watson Institute for International and Public Affairs at Brown University [4]
Not all events born from uncertainty are the same. For example, the Financial Crisis is different than Pearl Harbor, 9/11, and the Pandemic. Unlike the other unexpected events, the Financial Crisis was caused, in part, by the banking system itself, specifically the Mortgage Finance system. In the run-up to the financial crisis, the ability to anticipate the issues related to poor lending standards was clearly available. However, these lending issues were ignored and the Mortgage Finance system became destructively dysfunctional. We note this because the political will to “bail out the bankers” was clearly much higher during the Pandemic than it was during the Financial Crisis. In fact, the banking system itself was mobilized as a part of the Federal Government’s aid distribution system. This resulted from the CARES Act and related fiscal policies.
Be that as it may, all these unexpected events share some common ground. They are all characterized by an anticipatory failure of imagination and all share an outcome marked with significant uncertainty. Could we have anticipated the credit impact of an unexpected event? Possibly – most events born from uncertainty give rise to neglected prophets. For example:
- Prior to the Pandemic, Bill Gates described some of the growing risks associated with diseases. [5]
- Prior to the Financial Crisis, Raghuram Rajan, then a senior IMF official, warned about the growing risks in the financial system and proposed policies that would reduce such risks. [6]
However, following an uncertain event, this is mostly hindsight. Ideally, Financial Services companies forecast their credit losses in anticipation of an uncertain environment. There is good news for ESG, while there is uncertainty related to how and when ESG changes will impact financial services, there is an indication that ESG impacts WILL ultimately occur.
Tail Risk and Uncertainty in Action
Bankers and Credit Risk Managers tend to think in terms of Expected Loss or EL. This is an outcome-based on what we know from our latest credit data. We think in terms of a point estimate, which is at the high point of a probability distribution. The Unexpected Loss – UL or Stress Loss – is from the unknown. The further out in the tail we proceed, the more likely uncertain events will impact our data-enabled credit loss knowledge. As a practice, we make UL-based ACL adjustments regularly. We have an annual CCAR process to handle even bigger potential Stress Losses. But we still do not always get it right. The tails have become much thicker. This means the probability of the uncertainty associated with a tail risk event is higher than traditionally believed. This is a big deal. Paradoxically, “rare events” seem to be happening with some regularity. How we adapt to the unexpected is as much about company culture, scenario change process, and supporting technology as it is about picking the right scenario and the data analytics. The following are a few questions to evaluate a firm’s uncertainty and tail risk management environment:
- Does the firm have a governance process geared toward building and adapting uncertain scenarios?
- Does the firm have a process of properly weighing human judgment with past data?
- Does the firm have a process designed to a) encourage stakeholder buy-in, b) business updating and c) cultural adaptation to the new information? Does the firm invite people with unique knowledge to engage in scenario building?
- Does the firm use technology to facilitate scenario planning and prioritization?
With respect to uncertain events, credit loss estimation is in a challenging phase environment. In a very real sense, it is in a massive phase transition. Metaphorically, this transition can be related to physics, much like we think about matter phase change from ice to water, as heat is added. Think of uncertain events, like ESG or the pandemic, as heat that is driving an abrupt phase change, significantly impacting our credit loss understanding.
Credit loss estimation is also challenged by the nuanced but significant difference between risk and uncertainty. Particularly in recent years, credit risk policy and analytics require a VERY high reliance on historical data. Credit models are driven by historical information that, at its essence, describes human behavior. Simply put, the models describe the causal connection between HOW people behaved in the past and WHY they sometimes default on a loan in the future. But this data-rich risk world is periodically upended because of a sudden phase change.
The comfortable, data rich risk world is periodically replaced by the data limited uncertainty world. The uncertainty world is where historical data significantly lacks predictive power.
To be fair, the new uncertainty world is knowable, it is just not knowable in advance of the change. There will be some similarities to the old world but pretending the old credit models act as a loss point estimate foundation is a fallacy. Making a standard “On Top Adjustment (OTA)” to existing models should be met with skepticism. [7]
As an example of the credit modeling challenges, especially the use of Machine Learning (ML) and Artificial Intelligence (AI), follow this link to our article, The Inertia-On-Inertia Paradox. This paradox was especially evident in the last financial crisis. Model-based inertia was a driver of the Financial Crisis.
II) Simulation-Based Credit Analytics: The Credit Forecasting Under Uncertainty Approach
So, what is to be done about it? If the behavioral predictiveness of our data is suspect and a new phase state is in its infancy or unknown, how does one predict credit losses? That is, how are credit losses predicted on a book of loans created in the old risk-known world but unexpectedly transitioned into a new uncertainty-affected world?
The following suggests an approach to adjusting thinking and methods:
- Transition the thinking – stop pretending as if the old models work. If the words “On Top Adjustment” are being used to adjust for uncertainty, they should be discouraged. This is hard for us credit quants, we have invested years of work to interpret, engineer, accumulate, and cleanse data to do our job. It feels like a good friend passed away. There will be natural reluctance like a traumatic change. The imaginative thinking needed to manage uncertainty is an adjustment for those grounded in risk data.
- Consider the old, risk world models for what they are – while the new uncertainty environment may be dramatically different, core human behavior resulting from many millennia of evolutionary biology is relatively constant. People are incredibly consistent, resulting from: a) our cognitive biases, like our emotional influences related to fear and greed, b) how our brains flood our synapses with neurotransmitters, and c) our unchanging logical fallacies. Thus, people are still people. Behavioral models may no longer provide accurate credit loss point estimates. However, in some cases, they still rank order risk, especially in models where independent variables specific to human behavior are utilized. (Credit Bureau data is a good example.)
- Make bets on the new world – Now that the thinking is transitioning and the existing models are appropriately considered, it is time to implement a new forecasting method appropriate for the uncertainty world. This is uncomfortable for some that are accustomed to using deep data sets to build a unique perspective. These bets are structured with the assistance of Monte Carlo simulations (“sims”). That is, a new simulated world is modeled. The sim worlds are built on judgments of how people will respond in the new world, such as 1) what their buying behaviors are, 2) how unemployment impacts their ability to service current debt, 3) how the “reverse” wealth effect, as related to housing and security portfolio values, impact willingness to invest, 4) how long employment reallocation lasts and how it impacts different subsegments, 5) the length and degree of government support provided, etc. In effect, the simulation describes how the new world impacts the borrower’s ability to pay their existing “pre-change” originated loan. It also predicts how loan origination demand will adapt to the new world. It is possible that some of the old models could be appropriately utilized as inputs to the sims.
- Make multiple independent bets – In any situation with limited information, one would rarely make a single bet. The same is true in the new credit loss uncertainty world. Recognize, that there are multiple sim views directly related to the degree of uncertainty. To that end, multiple independent simulations should be created. Individual participants or small teams of credit-capable members should be assigned to the task. Please note: Having a single person or team performing the simulation is better than nothing. However, the accuracy of the forecast increases significantly as more credit-capable independent participants are engaged. Participant independence is important to drive a unique understanding of how the new world will play out. Also, multiple aggregated sample paths should be considered for each sim. That is, vary key assumptions based on outcome likelihoods. Each result should be considered, challenged, and utilized for a consensus. Frequent updating is important as the new world unfolds. As we learn more about the new world, expect the independent simulations to converge. [8]
A simulation approach for an uncertain world using environmental information and expert judgment. A retail lending example:
In support of the simulation approach, there is a rich literature base suggesting “improper linear models” can be just as good as linear regression models, and certainly better than models suffering from overfit or a lack of accuracy resulting from uncertainty. Per Robyn Dawes and other researchers, a set of independent variables, likely correlated to the dependent variable, with judgmentally set coefficients will likely perform quite well.
“The immediate implication of Dawes’s work deserves to be widely known: you can make valid statistical predictions without prior data about the outcome that you are trying to predict. All you need is a collection of predictors that you can trust to be correlated with the outcome.” [9]
The next section presents a simulation solution framework grounded in traditional lending decision techniques, known as the “Five Cs of Credit.” These judgmental techniques were regularly utilized prior to the heavy use of credit decision modeling today. The framework will assist the simulation participants to develop, as Dawes’ suggests, “a collection of predictors you can trust to be correlated with the outcome.”
III) Simulation-Based Credit Analytics: The Sim Solution
We continue with the retail lending example to provide a simulation-based solution. We utilize a simulation framework based on the time-tested “Five Cs Of Credit. In the past, banks relied on subjective judgment to assess the credit risk of a borrower. Today, much of that judgment has been codified in statistical and related decision models. Essentially, underwriters, credit analysts, and the decision models rely on the following borrower characteristic information in deciding whether to make a given loan. These characteristics are commonly referred to as the Five Cs of Credit [10], which are described in the table below.
Characteristic type is important as it describes the degree to which a characteristic is in direct control by the borrower.
Endogenous characteristics are unique to each borrower. The borrower has the direct ability to impact his or her endogenous characteristics.
Exogenous characteristics are generally outside of the borrower’s direct control, though it may impact the borrower’s probability of default (“PD”) or the loss given default (“LGD”). Exogenous characteristics generally refer to the macroeconomy.
To be fair, these characteristic types are not always strictly defined. For example, the collateral value will certainly have some exogenous impact from the macro environment. The point is that it was the borrower who ultimately made the endogenous choice of collateral and has control over its disposition.
Developing judgmental credit loss and related simulations are, by their very nature, a team sport. As such, the methodology must be collaborative, promote teamwork, and build consensus. It uses seven steps, as shown in the next graphic. Steps 1 and 2 are foundational. Once they are completed, the governance system and environmental data should be reassessed for continuous improvement on a periodic basis and in alignment with the operational steps. These foundational steps set the stage for the operational cycle of steps 3 through 7, which continues in an evolutionary fashion.
Next, the Monte Carlo scenario simulation segment and product build process are as follows:
Step 1: Establish the governance process
The governance process will be established. This may take the form of a Credit Loss Review Board (CLRB). The CLRB responsibility includes selecting products and associated resources to participate. The CLRB will approve the credentials of all those asked to participate. The Five Cs framework will be socialized and confirmed as the simulation framework for scenario planning. There are 4 different scenario segments based on 1) the individual borrower characteristics as captured in the endogenous factors, and 2) the likely macroeconomic changes captured in the exogenous market environment. (See the appendix for a scenario planning segmentation example.) The framework and process will be communicated to participants.
Step 2: Collect key environmental data
Data for each scenario segment and product will be collected using available information based on the organization’s borrower database information and market information from public and company licensed private sources. While participant independence is required for scenario modeling, base information should be shared across participants. As valuable participants, those members providing the data should also participate in the scenario planning exercise. Data that does not exist but is likely correlated to credit loss in the context of the uncertainty condition should be noted. This is a critical step as this is the information field from which all participants will create.
Step 3: Select key predictive credit drivers
The first step in the build stage is for each participant or team to familiarize themselves with the predictive credit drivers. At this point, participants will:
- Independently select a fixed number of credit drivers they believe to be correlated to credit risk and in the context of the uncertainty condition.
- Participate in a challenge session to consider additional data and to advocate for the participants’ data selection.
- Independently update the driver selection based on the veracity of the collective input.
At a minimum, one participant or team per segment and product is necessary. To increase the accuracy of the simulation, it is recommended to have multiple participants per segment.
Step 4: Weigh the power and interactions of credit drivers
Based on step 3, the participants will rate the relative importance and interactions of the credit loss drivers. This step is crucial to building an effective scoring model. The weighting factors must be accurate and enjoy the consensus of the group. The Analytic Hierarchy Process (AHP) is the gold standard for performing this critical step. [11] This step collects the judgment of the credit risk experts in an objective manner.
Step 5: Analyze the results
The facilitator-led credit loss scoring sessions generate a significant amount of data to analyze, requiring the generation of several charts and tables to support the analysis and facilitate the CLRB’s review and decision. The analysis covers strategic business value and profitability, credit loss and other performance driver-related costs, and strategic risks. The analysis approach enables the separation and clarification of objectives, facts, and the judgments of the team members.
Step 6: Optimize forecast based on scenario outcomes
The performance outcome simulates future losses and key performance measures 1 to 5 years forward. Additional estimates may be rendered via the simulation process, such as volume, attrition, and profitability. Note: the endogenous scenarios found in the appendix (1&2 or 3&4) are additive to provide a total loss estimate for the associated Exogenous Market Environment. Upon completion of the scenario segments, outcomes will be compared and utilized to drive the Allowance for Credit Loss or related performance estimating needs.
Step 7: Communicate and adapt the business to scenario outcomes
Prior to making business decisions, a sensitivity analysis is conducted to determine the degree to which the recommendations are sensitive to slight variations in the weighting of the credit loss drivers. The sensitivity analysis will increase the CLRB’s confidence in their decisions. The simulation is intended to be an ongoing process. Regularly, (at least annually) the simulation will be re-run. Ideally, some similar participants will be included, as well as “new blood” to bring a fresh perspective. Step 2’s environmental data will be updated and previously indicated “does not exist” data will be researched and provided as available.
The Key Benefits
It is important these sims are managed in a professional environment. This simulation decision-making environment consistently delivers the following benefits:
- Facilitating a professional sim process.
- Maintaining participant independence.
- Improved stakeholder engagement. including greater consensus and buy-in.
- Housing and presenting consistent background information to initiate the participant’s environmental understanding.
- Providing a straightforward, cloud-based workflow to capture the participant’s credit weighted scoring and other scenario planning factors.
- An optimization engine to evaluate simulated credit scenario outcomes.
- Reporting engine to summarize and assist in the communication of results.
- An ongoing, auditable, record to help maintain process governance and decision transparency.
- Enabling efficient scenario updating as new information is learned.
- Higher velocity decisions and better return on investment
IV. Conclusion
ESG, the Pandemic, the Russian invasion, and other events create significant credit uncertainty. The chaotic nature of a new uncertainty world creates significant differences in terms of how people respond and the changes related to their credit behavior. The article described why the new phase largely invalidates credit loss point estimates of the recent past and suggests an alternative credit loss estimation approach using Monte Carlo simulation modeling techniques.
Using a judgmental simulation model is appropriate given the lack of data available to drive desired decision support and as grounded in traditional credit decisioning. Process and technology support is necessary to implement the simulation approach. Ultimately, the new uncertainty world will give way to a more data-rich risk world. As this occurs, data will stabilize and grow, statistical confidence will increase, inertia will decline, and we will return to the comfortable world of risk modeling.
Until then, the Sim is our Friend
The Decision Support Solution Methodology was created by Definitive Business Solutions, Inc. This methodology is enabled by Definitive Pro®, a cloud-based, group decision support service that provides a collaborative process to build consensus and make complex, multi-criteria decisions in a wide range of scenarios. It uses the leading theory in multi-criterion decision making (the Analytic Hierarchy Process), which provides the ability to synthesize quantitative and qualitative factors and set priorities. It also employs a state-of-the-art mathematical programming solver to find the most favorable solution and optimize the allocation of resources. [XII]
- John Sammarco, President | JSammarco@DefinitiveInc.com
- Jeff Hulett, Executive Vice President | JHulett@DefinitiveInc.com
© 2022 Definitive Business Solutions. All Rights Reserved.
Appendix
This scenario planning example is for the Pandemic. It may be generalized for other uncertainty-based scenarios, like ESG or other uncertain events.
Notes:
[1] Roberta Wohlstetter, Pearl Harbor: Warning and Decision, June, 1962
[2] The 9/11 Commission Report at 9-11commission.gov, July 2004
[3] Wall Street and the Financial Crisis: Anatomy of a Financial Collapse, United States Senate Permanent Subcommittee on Investigations, April 2011
[4] Stephen Kinzer, The coronavirus pandemic is a failure of imagination, The Boston Globe, March 2020
[5] Bill Gates, Who Has Warned About Pandemics For Years, On The U.S. Response So Far, NPR, Heidi Glenn, April 2020.
[6] In 2005, Dr. Rajan said the financial system was at risk “of a catastrophic meltdown.”
[7] The subtle difference between risk and uncertainty is manifest when considering OTAs. Generally, making an OTA relates to potential qualitative events that may impact the risk-based expected loss, specific to its standard deviation. Uncertainty, on the other hand, is focused on tail risk, or kurtosis. Thicker tails may cause wildly different loss results, as per the Financial Crisis. Please see our article The numbers don’t lie – anticipating risk, managing uncertainty, and making quality decisions for more information.
[8] In general, using independent participants grounded approach to creating simulations is known as the “common task method.” Independent participants, utilizing common data sources compete to develop accurate outcomes. While this is not as common in credit loss estimation, it is common in computer science in the development of machine learning algorithms for standard tests or photograph interpretations. Other examples include (a) In 1980, Robert Axelrod, professor of political science at the University of Michigan, held a tournament of various strategies for the prisoner’s dilemma. He invited several well-known game theorists to submit strategies to be run by computers. (spoiler alert: a very simple “Tit For Tat” strategy won). b) In 2020, after a long-term, longitudinal study about “Fragile Families” was completed, a competition of over 160 teams was held to find the most predictive algorithms to answer key questions concerning teenage children at risk. Consistent with the game theory example, the simple models did quite well, almost as good as the more complex Machine Learning models. Given the danger of unrevealed overfit, one can argue simple is always better, especially when different algorithms have similar explanatory power. (To wit: Occam’s Razor)
[9] This quote is from the book Noise: A Flaw in Human Judgment by Daniel Kahneman, Olivier Sibony, Cass R. Sunstein.
[10] Troy Segal, The Five C’s Of Credit, March, 2020
[11] Thomas L. Saaty (1982) Decision Making for Leaders: The Analytic Hierarchy Process for Decisions in a Complex World
[12] Definitive Business Solutions, Inc. designed and integrated a bespoke solver engine, utilizing several solver programing code sets and data transmission capabilities, uniquely needed for the AHP and cloud environment.